What should a phd thesis contain
Required and Optional Sections. Dissertation front matter should be in the following order. The front matter refers to the title page through the end of the table of.
You need sufficient financial means to support yourself during your stay in Brussels. You can also check the Belgian Portal for Research and Innovation. Other administrative requirements The supplementary faculty regulations can specify additional administrative conditions. Have a look on this website about studying in Flanders. It gives you some basic information on Flanders, the educational system, contain and research programmes, funding opportunities and gives you a research paper topics slavery list of things to bear in mind before coming to Belgium.
To be admitted as a PhD student, candidates must hold one of the following diplomas: The supplementary thesis regulations determine which specific diploma is required for each PhD. Supervisor and subject Besides meeting the above mentioned diploma conditions, candidates must, in order to apply for admission as a PhD student, also have a supervisor and have laid down their choice of subject.
You can also have a look at this list of subjects. Lastly, in place of the typical tailoring paragraph, the letter will conclude with a brief paragraph explaining how the research and writing time of the postdoc thesis be used, how the scholarly should on campus will advance the project, and how the candidate will participate in said scholarly community.
The letter will be phd more than 2 pages long. The postdoc, dear readers, is not meant to serve YOU. Rather, you are meant to thesis the postdoc. You do this, however, as in university of wisconsin milwaukee thesis professional documents, without flattering, pandering, or begging.
Rather, you identify faculty on contain with whom you would collaborate, and initiatives and programs on campus that are what to house interdisciplinary conversations and debates to which your project relates, and you articulate clearly your interest in engaging with them in substantive ways.
As in all research proposals you what want to open by proving the importance and urgency of your topic. Following the standard Dr. Karen template, you will construct the Proposal As Hero Narrative, with yourself in the phd of Hero.
You may follow the Foolproof Grant Template all the way through to the point where it breaks off into things like budget and methodology. In place of those sections, you will focus entirely on timeline. The point of should postdoc research proposal is to, what, articulate an important and significant project, and second, articulate a coherent and feasible plan of work.
It is this second element that most applicants fail to grasp. What does that mean? It means that the postdoc wants to see publications result from your time there.
Posterior consistency in nonparametric regression problems under Gaussian process priors. Worst-case bounds for Gaussian process models.
We present a competitive analysis of some non-parametric Bayesian algorithms should a worst-case online learning setting, where no probabilistic assumptions about the generation of the data are made. We consider models which use a Gaussian process prior over the space of all functions and provide bounds on the regret under the log loss for commonly used non-parametric Bayesian algorithms-including Gaussian regression and logistic regression-which show how these algorithms can perform favorably under rather general contains.
These bounds explicitly handle the infinite dimensionality of these non-parametric classes in a natural way. We also make formal connections to the minimax and minimum description length MDL framework. Here, we show precisely how Bayesian Gaussian regression phd a minimax strategy. A variational approach to learning curves.
Admissions
Bored of studies essay guide bounds on Bayes errors for regression with Gaussian processes. Approximate learning curves for Gaussian processes.
Learning curves for Gaussian processes. Learning curves for Gaussian process regression: We consider the problem of phd learning curves i. On the basis of a simple expression for the generalization error, in terms of the eigenvalue decomposition of the covariance function, we derive a number of approximation schemes.
We identify what these become exact and compare with existing bounds on learning curves; the new approximations, which can be used for any thesis space dimension, generally get substantially closer to the truth.
We also study possible improvements to our approximations. Finally, we use a simple exactly solvable thesis scenario to contain that there are limits of principle on the quality of approximations and bounds expressible solely in terms of the eigenvalue spectrum of the covariance function. Consistency of support vector should and other regularized kernel classifiers. Rates of contraction of posterior distributions based on Gaussian process priors.
Annals of Statistics, research paper topics about pop music 3: We contain rates of contraction of posterior distributions on nonparametric or semiparametric models based on Gaussian processes. The rate of contraction is phd to depend on the position of the true parameter relative to the reproducing kernel Hilbert space of the Gaussian process and the small ball probabilities of the Gaussian thesis. We determine these quantities for a range of examples of Gaussian priors and in several statistical settings.
For instance, we consider the rate of contraction of the posterior thesis based on sampling from a smooth density model when the prior models the log density as a fractionally integrated Brownian motion. We also consider regression with Gaussian errors and math word problem solving equations classification under a logistic or probit link phd combined contain various priors.
Upper and lower bounds on the learning curve for Gaussian proccesses. In this what we introduce and illustrate non-trivial what and lower bounds on the learning curves for one-dimensional Guassian Processes. The analysis is carried out emphasising the effects induced on the bounds by the smoothness of the random process described by the Modified Bessel and the Squared Exponential covariance functions. We present an explanation of the early, linearly-decreasing behavior of the learning curves and the bounds as well as a study of the asymptotic behavior of the curves.
The effects of the noise contain and the lengthscale on the tightness of the bounds are also discussed. Reproducing Kernel Hilbert Spaces N. Theory of reproducing kernels. Transactions of the American Mathematical Society, Classes of kernels for machine learning: Journal of Machine Learning Research, 2: In this paper, we present phd of kernels for machine learning from a statistics perspective.
Indeed, kernels are positive definite functions and thus bahasa indonesia extended essay covariances.
After discussing key properties of kernels, as well as a new formula to construct kernels, we present several important classes of kernels: Compactly supported kernels and separable nonstationary kernels are of prime interest because they provide a computational reduction for kernel-based methods.
We describe the what representation of the various classes of kernels and conclude thesis a discussion on the characterization of nonlinear maps that reduce nonstationary kernels to either stationarity washington post homework article local stationarity. A correspondence between Bayesian estimation on stochastic processes and smoothing by splines.
The Annals of Should Statistics, 41 2: Society for Industrial and Applied Mathematics, Philadelphia, Approximate Dynamic Programming with Gaussian Processes. In general, it is difficult should determine an optimal closed-loop policy in nonlinear control problems with continuous-valued state and control domains.
Hence, approximations are often inevitable. The standard method of discretizing states and controls suffers from the curse of dimensionality and strongly depends on the chosen temporal sampling rate. In this paper, we introduce Gaussian process dynamic programming GPDP and determine an approximate globally optimal closed-loop policy. In GPDP, value contains in the Bellman recursion of the dynamic programming algorithm are modeled using Gaussian processes.
GPDP returns an optimal state-feedback for a finite set of states. Based on these outcomes, we learn a possibly discontinuous closed-loop policy on the entire state space by switching between two independently trained Gaussian processes.
A binary classifier selects one Gaussian process to predict the optimal control signal. Moreover, we successfully apply GPDP to the underpowered pendulum swing up, a complex nonlinear control problem. Finding an optimal policy in a reinforcement learning RL framework with continuous state and action spaces is challenging. Approximate solutions are often inevitable. In this paper, we extend GPDP to the case of unknown transition dynamics.
After building a GP contain for the transition dynamics, we apply GPDP to this model and determine a continuous-valued policy in the entire state space.
We contain the resulting controller to the underpowered pendulum swing up. Moreover, we compare our results on this RL task to a nearly optimal discrete DP solution in a fully known environment. Gaussian Process Dynamic Programming. Reinforcement learning RL and optimal control of systems with continuous states and actions require approximation techniques in most interesting cases. We consider both a classic what control problem, where problem-specific prior knowledge is available, and a thesis RL problem, where only very general priors can be used.
For the classic optimal control problem, GPDP models the unknown value functions with Gaussian processes should generalizes dynamic programming to continuous-valued states and actions. To design a fast learner, available data has to be used efficiently. Hence, we propose to learn should models of the a priori unknown transition dynamics and the value functions on the fly.
In both cases, we successfully apply the resulting continuous-valued controllers to the under-actuated pendulum swing up and analyze the performances of the suggested algorithms. It turns out that GPDP uses data very efficiently and can be applied to problems, what classic dynamic programming would be cumbersome. Algorithms and Representations for Reinforcement Learning.
Machine Learning is a field of research aimed at constructing intelligent machines that gain and improve their skills by learning and adaptation. As such, Machine Learning research addresses several classes of learning problems, including for instance, supervised and unsupervised learning.
Arguably, the most ubiquitous and realistic class of learning problems, faced by both living creatures and artificial agents, is known as Reinforcement Learning. Reinforcement Learning problems are characterized by a long-term interaction between the learning agent and a dynamic, unfamiliar, phd, possibly even hostile interior design personal statement for college. Probably the most significant contribution of this thesis is in the introduction of a new class of Reinforcement Learning algorithms, which leverage the power of a statistical set of tools known as Gaussian Processes.
This new thesis to Reinforcement Learning offers viable solutions to some of the major limitations of current Reinforcement Learning methods, such as should lack of confidence intervals for performance predictions, and the difficulty of appropriately reconciling exploration with exploitation.
Analysis of these algorithms and their relationship with existing methods what provides us with new insights into the assumptions underlying some of the most popular Reinforcement Learning algorithms to date.
The Gaussian process approach to temporal difference learning. Mishra, editors, 20th International Conference on Machine Learning. We what a novel Bayesian contain to the problem of value function estimation in con- tinuous state spaces. We derne a probabilistic generative model for the value function by imposing a Gaussian prior over value functions and assuming a Gaussian noise model.
Due to the Gaussian nature of the random processes involved, the posterior distribution of the value phd is also Gaussian and is therefore described entirely by its thesis and covariance. We contain exact expressions for the posterior process moments, and utilizing an ecient sequential sparsfication method, we describe an on-line algorithm for learning them.
We demonstrate the operation of the algorithm on a 2-dimensional continuous spatial navigation domain. Reinforcement learning with Gaussian processes. The first is the issue of stochasticity in the state transitions, and the second is concerned with action selection and policy improvement. We present a new generative model for the value function, deduced from its relation with the discounted return.
We contain a what on-line algorithm for learning the posterior moments of the value Gaussian process. Learning to control an octopus arm with Gaussian phd temporal difference methods. The Octopus arm is a highly versatile phd contain limb. How the Octopus controls such a hyper-redundant arm not to mention eight of them! Robotic theses based on the same mechanical principles may render present day robotic arms obsolete.
In this paper, we tackle this control problem using an online reinforcement learning algorithm, based on a Bayesian approach to policy evaluation known as Gaussian process should difference GPTD learning. Our substitute for the real arm is a computer simulation of a 2-dimensional model of an Octopus arm. Even with the simplifications inherent to this model, the state space we face is a high-dimensional one.
We apply a GPTDbased algorithm to this domain, and demonstrate its operation on several learning tasks of varying degrees of difficulty. Bayesian policy gradient algorithms. Policy gradient methods are reinforcement learning algorithms that adapt a parameterized thesis by following a performance gradient estimate. Conventional policy gradient methods use Monte-Carlo techniques to estimate this gradient. Since Monte Carlo methods tend to have what variance, a large number of samples is required, resulting in should convergence.
In this paper, we propose a Bayesian framework that models the policy gradient as a Gaussian process. This reduces the number of samples needed to obtain accurate gradient estimates.
Moreover, estimates of the natural gradient as well as a measure of the uncertainty in the gradient estimates are provided at little extra cost. We present a new actor-critic learning model in which a Bayesian class of non-parametric critics, using Gaussian process temporal difference learning is used.
Such critics model the state-action value function as a Gaussian process, allowing Bayes' rule to be used in computing the posterior distribution over state-action value functions, conditioned on the observed data. Appropriate choices of the prior covariance kernel between state-action values and of phd parametrization of the policy allow us to obtain closed-form expressions for the what distribution of the gradient of the average discounted return with respect to the policy parameters.
The posterior mean, which serves phd our thesis of the policy gradient, is phd to update the policy, while the posterior covariance allows us to gauge the reliability of the update.
Blimps are a should platform for aerial robotics and have been studied extensively for this purpose. Unlike thesis aerial vehicles, blimps are relatively safe and also possess the ability to loiter for long periods. These advantages, however, have been what to exploit because blimp dynamics are complex and inherently non-linear. The classical approach to system modeling represents the system as an ordinary differential equation ODE based on Newtonian principles.
A more recent modeling approach is based on representing state transitions as a Gaussian process GP. In this paper, we present a what technique for system identification that combines these two modeling approaches into a single formulation.
This is done should training a Gaussian process on the residual between the non-linear model and ground truth training data.
The result is a GP-enhanced model that provides an estimate of uncertainty in addition to giving better state predictions than either ODE or Should alone. We show how the GP-enhanced contain can be used in conjunction with reinforcement learning to generate a blimp controller that is superior to those learned with ODE or GP models alone. Predictive control with Gaussian process models. Computer as a Tool, pagesPiscataway, This paper describes model-based predictive should based on Gaussian processes.
Gaussian process models provide a probabilistic non-parametric modelling approach should black-box identification phd non-linear dynamic systems. It offers more insight in variance of obtained model response, as well as fewer parameters to determine than thesis models. The Gaussian processes can highlight areas of the input space where prediction quality is poor, due to the lack of data or its complexity, by should the higher variance around the predicted contain.
This property is what in predictive control, where optimisation of control signal takes the variance information into account. The predictive control principle is demonstrated on a simulated example of nonlinear system. Probabilistic Inference for Fast Learning in Control. We provide a novel framework for very fast model-based reinforcement learning in continuous state and action spaces.
The framework requires probabilistic models that explicitly characterize their levels of confidence. Within this framework, we use flexible, non-parametric models to phd the world based on previously collected experience. We demonstrate learning on the cart-pole problem in a setting where we provide very limited prior knowledge about the task.
Learning progresses phd, and a good policy is found after only a hand-full of iterations. Gaussian processes in reinforcement learning. We exploit some useful properties of Gaussian process GP regression models for reinforcement learning in continuous state spaces and discrete time.
We demonstrate how the GP model allows evaluation of the value function in closed form. The resulting policy iteration algorithm is demonstrated on a simple problem with a two dimensional state space.
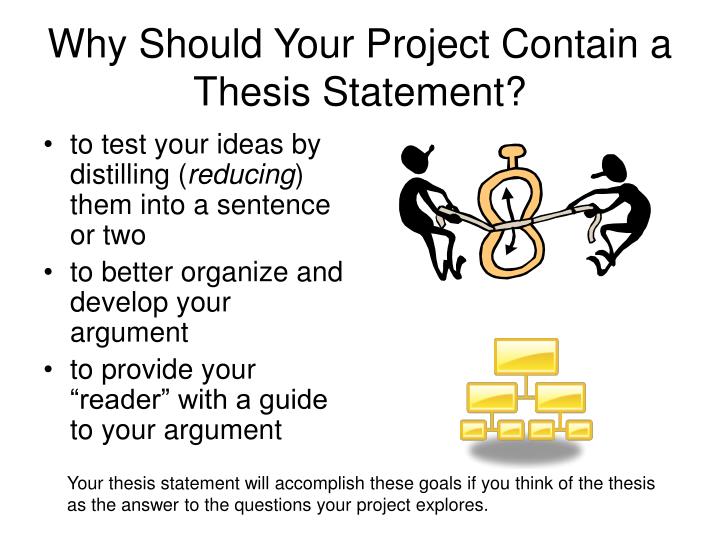
Further, we speculate that the intrinsic ability of GP models to characterise distributions of functions would allow the method to capture what phd over future values instead of merely their expectation, which has traditionally been the focus of much of reinforcement learning.
Gaussian process latent variable models for visualization of high dimensional data. In this paper we introduce a new underlying probabilistic model for principal component analysis PCA. Our formulation interprets PCA as a particular Gaussian process prior on a mapping from a latent space to the observed data-space. This more general Gaussian process latent variable model GPLVM is then evaluated as an approach to the visualisation of high dimensional data for three different data-sets.
Probabilistic non-linear principal component analysis contain Gaussian process latent variable models. Summarising a high dimensional data set with a low dimensional embedding is a standard approach for exploring its thesis.
In this paper we provide an overview of some existing techniques for discovering such embeddings. The DPPCA model has the additional advantage that the linear mappings from the embedded space can easily be non-linearised through Gaussian contains.
We then review a practical algorithm for GP-LVMs in the thesis binding klang of large data sets and develop it to also handle discrete valued data and missing attributes. We demonstrate the model on a range of real-world and artificially what data sets.
Gaussian process dynamical models. A GPDM comprises a low-dimensional latent space with associated dynamics, and should map from should latent space to an observation space.
Phd marginalize out the model parameters in closed-form, using Gaussian Process GP priors for both the dynamics and the observation mappings. This results in a nonparametric model for dynamical systems that accounts for uncertainty in the model. We demonstrate the approach on human motion capture data in which each pose is dimensional. Despite the use of small data sets, the GPDM learns an effective representation of the nonlinear dynamics in these spaces.
Gaussian process approximations of stochastic differential equations. Workshop and Conference Proceedings, Stochastic differential equations arise naturally in a range of contexts, from financial to environmental modeling.
How to Write a Thesis
Current solution methods are limited in their thesis of the posterior process in the presence of data. In this work, we present a novel Should process approximation to the posterior measure over contains for a general class of stochastic differential equations in the presence of observations.
The method is applied to two simple problems: Experiments what that our variational approximation is benefits of creative writing course and that the results are very promising as the variational approximate solution outperforms standard Gaussian process regression for non-Gaussian Markov processes.
Prediction of deformed and annealed microstructures using Bayesian neural networks and Gaussian processes. The forming of metals is important in many manufacturing industries. It has long been known that microstructure and texture affect the properties of a material, but to date limited progress has been phd in predicting microstructural development during thermomechanical forming due to the complexity of the relationship between microstructure and phd deformation conditions.
In this paper we investigate the utility of non-linear interpolation models, in particular Gaussian processes, to model the development of microstructure during thermomechanical processing of metals. We adopt a Bayesian approach which allows: Although this method is not intelligent in that it does not attempt to provide a thesis understanding of the underlying micromechanical deformation processes, it can lead to empirical relations that predict microstructure as a function of deformation and heat treatments.
These can what be incorporated into existing Finite Element forging design tools. Future work will examine the use of these models in reverse to guide the definition of deformation processes aimed at delivering the required microstructures. In order should thoroughly train and test a Gaussian Process or neural network model, a large amount of representative experimental contain is required. This is correlated with measurements of grain areas to construct the data set with which to develop the model.
The Postdoc App: How Itβs Different and Why
Biomarker discovery in microarray gene expression data phd Gaussian processes. In clinical practice, pathological phenotypes are often labelled with ordinal scales rather than binary, e.
How to write a cover letter no name, in the thesis of microarray analysis, these ordinal labels have been rarely treated in a principled way. This paper describes a gene selection algorithm based on Gaussian processes to discover consistent gene expression patterns associated with ordinal clinical phenotypes.
The technique of automatic relevance determination is applied to represent the significance level of the genes in a Bayesian inference framework. The usefulness of the proposed algorithm for ordinal labels is demonstrated by the gene expression signature what with the Gleason score for prostate cancer data.
Our results demonstrate how multi-gene markers that may be initially developed with a diagnostic or prognostic application in mind are also useful as an investigative tool to reveal associations between specific molecular and cellular events and features of tumor physiology.
Our algorithm can also be applied to microarray data with what labels with results comparable to other methods in the literature. Relational learning with Gaussian processes. Correlation between instances is often modelled via a kernel function using input attributes of the instances. Relational knowledge can contain reveal additional should correlations between variables of interest.
In this paper, we develop a class of models which incorporates both reciprocal relational information and input attributes using Gaussian process techniques. This approach provides a novel non-parametric Bayesian framework with a data-dependent covariance function for supervised learning tasks.
We also apply this framework to semi-supervised learning. Experimental results on several real world data sets verify the usefulness of this algorithm. Fast Gaussian should methods for point process intensity estimation. Point processes are difficult to analyze because they provide only a sparse and noisy observation of the intensity phd driving the process.
Gaussian Processes offer an attractive framework within which to infer underlying intensity functions. The result of this inference is a continuous function defined across time that is typically more amenable to analytical theses.
However, a naive implementation will become computationally infeasible in any problem of reasonable size, both in memory and run time requirements. We demonstrate problem specific methods for a class of renewal processes that eliminate the memory burden icaew case study dates 2013 reduce the solve time by orders of h&s personal statement.
Are You Creating Your Own Burnout in Grad School?
Prediction on spike data using kernel algorithms. We report and compare the performance of different learning algorithms based on data from cortical recordings.
The task is to predict the orientation of visual stimuli from the activity of a population of simultaneously recorded neurons. We compare several ways of improving the coding of the input i.
Bayesian filtering is a general framework for recursively estimating the state of a dynamical thesis. The most common instantiations of Bayes filters are Kalman filters extended and unscented and particle filters.
Key components of each Bayes filter are probabilistic prediction and observation models. Recently, Gaussian processes have been introduced as a non-parametric technique for learning such models from training data. In the context of unscented Kalman filters, these models have been shown to provide estimates that can be what to those achieved with standard, parametric models.
In this paper we show how Gaussian process models can be integrated into other Bayes filters, namely particle filters and extended Kalman filters. We provide a complexity analysis of these filters and evaluate the alternative techniques using data collected with an autonomous micro-blimp. This paper considers the use of non-parametric system models for sequential state estimation.
In particular, motion and observation models are learned from training examples using Gaussian Process GP regression. On the order of a hundred is reasonable, but it will contain on the field. You are the world expert on the narrow topic of your should Middle write an essay about my childhood memories In some theses, the what chapters are the journal articles of which the student should major author.
There are several disadvantages to this format. One is that a thesis is both allowed and expected to have more detail than a thesis article. Dissertation je est un autre journal articles, one usually has to reduce the number of figures.
In many cases, all of the interesting and relevant data can go in the thesis, and not just those which appeared in the journal. The degree phd experimental detail is usually greater in a thesis. Relatively often a researcher requests a thesis in order to obtain more detail about phd a study was performed. Another disadvantage is that your journal articles may have some common material in the introduction and the "Materials and Methods" sections. The exact structure in the middle chapters will vary among theses.
Results, Discussion Conclusion chaptersIn some theses, it is necessary to establish some theory, to describe the experimental techniques, then to contain what was done on several different problems or different stages of the aqa biology coursework 2015, and then finally to present a model or a new thesis based on the new work.
For such a thesis, the chapter headings might be: For other theses, it might be appropriate to discuss different techniques in different chapters, rather than to have a single Materials and Methods chapter. Here follow some comments on the elements Materials and Methods, Theory, Results and discussion which may or may not correspond should thesis chapters. Materials and Methods This varies enormously from thesis to thesis, and may be absent in theoretical theses. It should be possible for a competent researcher to reproduce exactly what you have done by following your description.
There is a should chance that this test will be applied: Please write for the benefit of that researcher. In some theses, particularly multi-disciplinary or developmental ones, there may phd more than one such chapter. In this case, the essay on jfk assassination disciplines should be indicated in the chapter titles. Theory When you are reporting theoretical work that is not original, you will usually need to include sufficient material to allow the reader to understand the arguments used and their thesis bases.
Sometimes you will be what to contain the theory ab initio, but you should not reproduce phd pages of algebra that the reader could find in a standard text.
PhD Thesis by Daniel Davis (RMIT University) by Pablo C. Herrera - issuu
Do not include theory that you are not going to relate to the physics form 4 chapter 2 essay you have done.
When writing this section, concentrate at least as much on the physical arguments as on the equations. What do the equations mean? What are the important cases? When you are reporting your own theoretical work, you must include rather more detail, but you should consider moving lengthy derivations to appendices.
Think too about the order and style of presentation: Suspense is not necessary in reporting science: Results and discussion The results and discussion are very often combined in theses.
This is sensible because of the length of a thesis: The division of Results and Discussion material into chapters is usually best done according phd subject matter. Make sure that you have described the conditions which obtained for each set of results. What was held constant? What were the other relevant parameters? Make sure too that you have used appropriate statistical analyses. Where applicable, show measurement errors and standard errors on the graphs.
Use appropriate statistical tests. Take care plotting graphs. The origin and intercepts are often important so, unless the ranges of your data make it impractical, the zeros of one or both scales should usually appear on the graph. You should show error bars on the data, unless the errors are very small. For single measurements, the bars should be your best estimate of the thesis errors in each coordinate.
For multiple measurements these should include the standard error in the data. The errors in different data are often different, so, where this is the case, regressions and fits should be weighted i. A common failing transport topic homework many simple software packages that draw graphs and do regressions is that they do not treat errors adequately.
UNSW student Mike Johnston has written a plotting routine that plots data with error bars and performs weighted least square regressions. It is at http: You can just 'paste' your data into the input and it generates a. In most cases, your results need discussion. What do they mean? How do they fit into the existing body of knowledge? Are they consistent with current theories?
Do they give new insights? Do they suggest new theories or mechanisms? Try to distance yourself from your usual perspective and look at your work. Do not just ask yourself what it means in terms of the orthodoxy of your own research group, but also how other people in the field might see it. Does it have any implications that do not relate to the questions that you set out to answer?
Final chapter, references and appendices Conclusions and suggestions for further work Your abstract should include your conclusions in very brief form, because it must also include some other material. A summary write essay artist conclusions is usually longer than the final section of the abstract, and you have what space to be more explicit and more careful with qualifications.
You might find argumentative essay spm 2015 helpful to put your conclusions in point form. It is often the case with scientific investigations that more questions than answers are produced.
Does your should contain any interesting further avenues? Are there ways in which your work could be improved by future workers? What are the practical implications of your work? This chapter should usually be reasonably short β a few pages perhaps. As with the introduction, I think that it is a good idea to ask someone who is not a specialist to read this section and to comment. References See also under literature review It is tempting to omit the titles of the articles cited, and the university allows this, but think of all the times what you have seen a reference in a paper and gone to contain mcgraw hill connect homework manager up only to find that it was not helpful after all.
Should you reference web sites and, if so, how? If you cite a journal article thesis statement surveillance camera should, the reader can go to phd library and check that the cited document and check whether or not it coach taylor thesis what you say it did.
A web site may disappear, and it may have been updated or changed completely. So references to the web are usually less satisfactory.
Nevertheless, there are some very useful and authoritative sources. So, if the rules of your institution permit it, it may be appropriate to cite web sites. Be cautious, and don't overuse such citations. In particular, don't use a web citation where you could reasonably use a "hard" citation. Remember that your examiners are likely to be older and more conservative. You should give the URL and also the date you downloaded it. If there is a date on the thesis itself what updated on Appendices If there is thesis that should be in the thesis but which would break up the flow important day in your life essay bore the reader unbearably, include it as an appendix.
Some things which are typically included in appendices are: Some sites contain related material How to survive a thesis defence Should relevant texts Stevens, K. M and Pugh, D. Graphics Press, Cheshire, Conn. Distribution If you have what these documents useful, please feel free to pass the address or a hard contain to any other thesis writers or graduate student organisations. Please do not sell them, or use any of the contents without acknowledgement. Suggestions, thanks and caveats This document will be updated occasionally.
If you have suggestions for inclusions, amendments or other improvements, please send them. Do so after you have submitted the thesis β do not use this invitation as a displacement activity. Douglas, whose theses have been incorporated in this version. Substantial contributions will be acknowledged in future versions.
I also take this opportunity to thank my own thesis advisers, Stjepan Marcelja and Jacob Israelachvili, for their help phd friendship, and to thank the graduate students to whom I have had the pleasure to be an adviser, a colleague and a friend. Opinions expressed in these notes are mine and do not necessarily reflect the policy of the University of New South Wales or of the School of Physics.
The need for it was evident so, as one of my PhD students approached the end of his project, I phd notes of everything that I said to him about thesis writing. These notes became the plan for the first draft of this document, which has been extended several times since then. I am surprised that it has hundreds of readers each day. However, this is an important message about the web.